Test-before-invest Experiments
The Test-Before-Invest experiments within the AI REDGIO 5.0 project are organized into three distinct categories, each designed to address key aspects of AI integration in manufacturing:
-
Technology and Regulatory Sandboxes for AI (TERESAs): These experiments focus on the validation of AI solutions that involve human-machine interaction and human-in-the-loop components. They also examine the legal, ethical, and human-centric dimensions of AI technologies, ensuring responsible deployment and compliance with regulatory standards in industrial settings.
-
Virtual Factory Experiments (VFs): Utilizing virtual assets from the didactic factories, these experiments explore the role of digital twins and virtual simulations in optimizing manufacturing processes. They aim to enhance efficiency, improve real-time monitoring, and support data-driven decision-making, showcasing the power of virtual environments in modern manufacturing.
-
Didactic Factories (DF Experiments): These experiments test and refine AI tools in real-world scenarios, aiming to optimize and validate their practical application. They demonstrate the effectiveness and impact of AI in improving industrial processes, enabling more efficient and sustainable operations.
Together, these experiments form a comprehensive framework within the AI REDGIO 5.0 project, advancing AI technology in manufacturing through technical validation, ethical considerations, and hands-on application, while promoting sustainable and human-centric solutions.
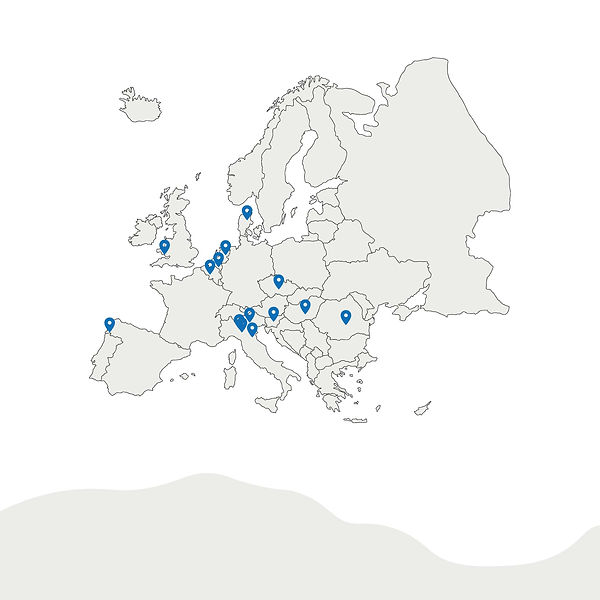
Test-before-invest experiments
Industry4.0Lab
Experiment description:
Lombardy Industry4.0Lab at POLIMI is a Didactic Factory which since 2016 has been used to exploit relevant research outcomes into real manufacturing environment. The Didactic Factory consists of a semi-automated assembly line which simulates the production flow of electronic devices and of additional robotic assets, such as two cobots and an AGV which project the didactic experience towards the future trends of manufacturing.
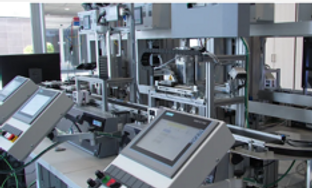
The experiment will make the lab software architecture more decentralized and resilient thanks to the installation of new hardware directly on the machine. This will substitute the existing one, limited to the gathering, filtering and formatting of raw data, to make the system more resilient towards unexpected events and more sustainable in environmental terms, as the data processing will be moved on the more efficient edge layer.
Experiment description:
ACTEMA (Advanced Control and Technologies for Enhanced Mechatronics and Automation) is the Didactic Factory managed and operated by the ACTEMA Research Group of the DEI (Department of Electrical, Electronic and Information Engineering "Guglielmo Marconi"), of the University of Bologna. ACTEMA develops methods and technologies in the field of control, monitoring, and diagnosis of innovative mechatronic and automation systems.

The main goal of the experiment is to develop an edge solution, from hardware and software point of view, with algorithm for predictive maintenance. The edge solution is a key point to divide the overall computational algorithms in two main level: in edge computer solution, and a remote solution on or on premises. The key part of experiment is to design a build on a new edge computing, new features from algorithms.
IJS Systems & Control Lab
Experiment description:
Didactic factory IJS Systems & Control Lab at the Department of Systems and Control (JSI) is an applied R&D facility that consists of several sets of demonstrations of industrial system and hydrogen technologies. The systems are dedicated to support the development and testing of new green and digital technological solutions and to promote their wider applicability and usability.

The experiment “Self-evolving monitoring systems for assembly production lines” consists of an industrial set-up representing a part of the DC drive assembly process. The experiment will set up a testbed for self-evolving solutions for monitoring production asset performances, targeting repetitive processes, such as assembly production lines. Demonstrated diagnostic systems will self-learn by analyzing the patterns from process time-series measurement, while using machine-learning methods.
Flexible Manufacturing Field Lab
Experiment description:
Flexible Manufacturing Field Lab at BPI Brainport Innovation Campus in Eindhoven is a lab which concentrates on flexible production and assembly solutions, while also focuses on robotization of manufacturing processes. Moreover, the BIC hosts Multi Material 3D where research pilots on automation for multi-industry 3D applications take place. Also, a field lab called High Tech Software Cluster houses with the focus on AI, ML, Big Data Analytics, Data Services, Industrial 5G, Industrial IOT, and Wireless Communication.
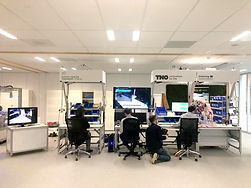
The experiment focuses on generating synthetic datasets that are statistically meaningful and reflect real data in ways that are relevant to yield improvement. Most recently, AI and machine learning algorithms have been used to create synthetic data that is more useful and representative and are used to train and calibrate models before real-world data becomes available.
Advanced Manufacturing Center (AMC)
Experiment description:
The Advanced Manufacturing Center (AMC) will be the physical embodiment of the Fraunhofer Project Center (FPC) at the University of Twente in Enschede (the Netherlands). AMC is an open innovation center for digital transition in manufacturing, developing pathways towards smarter industry, whose core activities consist of application-oriented research, demonstrators, and knowledge transfer: (i) Demonstration and showcasing; (ii) Test before invest; (iii) Prototyping; (iv) Provision of training platform.
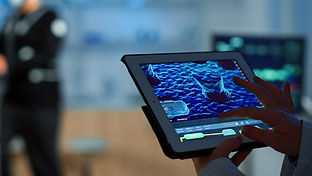
Environmental sustainability through AI is the key topic of the experiment. A full-scale instrumentation will be available as well as appropriate digital infrastructure(s) bringing together data from different sources to eventually built-up digital twins. Dedicated innovative solutions to address environmental targets will be developed and demonstrated.
4.0iLab
Experiment description:
4.0iLab at Fondazione Bruno Kessler (Trentino) is a facility created to support tech transfer activities (co-design, prototyping and technological integration of 3rd-party solutions) of IoT sensing applications, cognitive techniques and advanced ICT solutions for the control of connected machines and plants and monitoring of industrial environments. The FBK 4.0iLab is functional for the realization of prototypes, in the context of IoT and AI-based industrial applications.

The experiment will deal with specific topics of work based on Digitalisation/AI used to reduce costs/increasing revenue according to a circular economy approach, such as quality monitoring in the whole production process, predictive maintenance, product traceability, management of industrial by-products.
Predictive Maintenance 5.0 Didactic Factory (PM50)
Experiment description:
Flanders Make’s existing Predictive Maintenance 5.0 Didactic Factory (PM50), located at the Leuven (Belgium), consists of a fleet of seven drivetrain setups with safety casing and with high frequency vibration, T, load, and speed sensors with on-the-fly AI-based processing of raw data by a central computer for predictive maintenance. It is used for accelerated lifetime tests of bearings and to guide maintenance technicians in product lines to get acquainted with new technologies.

The experiment consists of upgrading the existing DF concerning Predictive Maintenance from Industry 4.0 to Industry 5.0, with AI on the edge and easy-to-use and focused human-machine interfacing for decision support and providing dissemination events so that engineers of SMEs can experience I5.0.
Digital Manufacturing Innovation Hub Wales (DMIW)
Experiment description:
The Digital Manufacturing Innovation Hub Wales DMIW is a demonstration of the latest Industry 4.0 technologies including PLC and control systems connectivity, cloud-based data capture, AI Vision Systems, haptic feedback, CPS and embedded technologies, gamification and multi-vendor integration across a range of digital and analogue sensors.
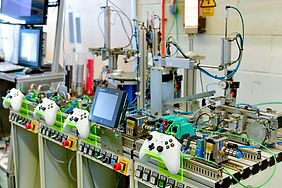
The purpose of this experiment is to take a typical production scenario, such as an assembly process, and use the already available data to create a stream of information which the IWOK system can use to suggest a solution to a problem to the Operator, who can in turn use their ‘human’ I/O to verify the solution and ‘teach’ the machine how to fix the problem itself. This then creates a living Knowledge base between the Operator and the Machine that can be used to avoid problems before they occur.
MADE Competence Center Industry 4.0
Experiment description:
MADE is an Italian Competence Center, acknowledged as Digital Innovation Hub by EU. It aims at supporting manufacturing digital and sustainable transformation providing a set of knowledge, methods, technical and managerial skills on digital technologies. MADE provides 6 preindustrial manufacturing scenario and 25 interoperable use cases for pioneering test, demonstration and pilot project development.
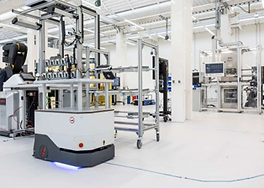
BEhAI is a DF driven experiment in Lombardy focused on implementing AI inside manufacturing. What the experiment wants to demonstrate is how the application of the AI linked to sensors’ data can enhance human-machine interactions by identifying the state of the operator and adjust its patterns and functions to it, accordingly.
This will be done to address two main challenges:
- Understand and adapt to human states;
- Understand and adapt to context.
TUIASI didactic factory
Experiment description:
The TUIASI didactic factory models Industry 4.0 innovative aspects of a production plant aiming to provide hands-on and interactive research and training in applications of advanced technologies. Its main components are: (i) autonomous omnidirectional robots; (ii) artificial vision systems; (iii) collaborative manipulator; (iv) 3D printed electronics manufacturing system.
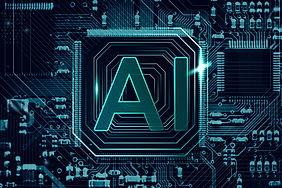
QUAD-AI@E experiment will develop a product defect detection system able to acquire product images, to manipulate images by an embedded AI tool, to extract info about the nature and severity of the product defect and to send alert to the personnel. The experiment builds on integrating AI at the edge technology into a product defect detection system prototype suitable for quality control automation in textile industry.
Testbed for Industry 4.0
Experiment description:
Testbed for Industry 4.0, as part of the EU Centre of Excellence RICAIP (Research and Innovation Centre on Advanced Industrial Production), represents the first multi-site/distributed research and experimental manufacturing facility in Europe for advanced industrial production with a powerful supercomputer for AI research and applications, 4 separate laboratories for measuring and solving transport and logistics issues, robot vision workstation, etc.
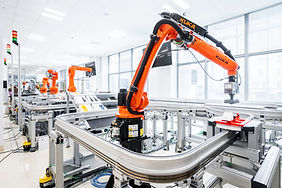
The experiment aims to demonstrate an AI-driven real-time monitoring of a robotic assembly line equipped with a delta robot assembling a small 4-wheel vehicle. The core problem of the monitoring is the detection of non-OK state from force and torque time series acquired by sensors positioned on the robot end effector. The main goal is to detect a problem quickly after it appears so that a corrective action will come early, which can significantly improve the assembly process. The problem is formulated as a real-time anomaly detection from multidimensional time-series, which can be efficiently solved by machine learning techniques.
Experiment description:
The AAU Smart Lab at Aalborg University is "small Industry 4.0 factory” integrating and demonstrating Industry 4.0 technologies, built around FESTOs CP factory, which is a small modular, and expandable factory integrating many relevant Industry 4.0 technologies (e.g. IIoT, AI, Robotics, simulation, digital twin, clouds/edge, 5G).
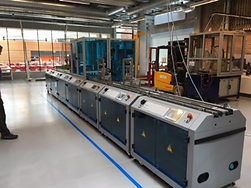
The experiment will update the AAU IoT suitcase to include more advanced data analytics and machine learning capabilities. One machine type from the host SME will be selected and used as basis for experimenting and validating data-driven technologies.
am-Lab Teaching and Learning Factory
Experiment description:
The am-Lab Teaching and Learning Factory unit at PBN focuses on digitalization in manufacturing, in 4 technological domains – robotics, data science, extended reality and 3D technologies. All relevant technologies are available at in house. Recently, a 5-module TLF was installed and used as a platform to integrate the existing digitalization tools – connecting to mobile robot, real-time sensor data visualization, etc.

PBN am-Lab in Hungary is conducting the SUNSYNC experiment, aiming to optimize recycling processes in industrial environments. The experiment focuses on automating and streamlining recycling methods, utilizing solar power as the primary energy source, extending battery life, and making data-driven decisions throughout the project. The experiment involves implementing an AI-driven system that integrates data from light detectors, weather forecasts, and accumulator monitoring systems. The process includes data integration, AI-based data processing, and real-time decision-making to optimize energy use and align recycling operations with available clean energy.
Didactic Factory in Galicia
Experiment description:
The Didactic Factory in Galicia is located at AIMEN's facilities, which are equipped with state-of-the-art automation and robotics technologies to provide advanced and digital manufacturing solutions for various industries. It aims to support and boost the local manufacturing industry (mainly automotive, naval and food industry) by serving as a hub for research and development in these domains to students and local companies. To this end, with the collaboration of Gradiant, it plans to integrate diverse cutting-edge technologies, such as AI, 5G, cloud-edge continuum, online learning, etc.
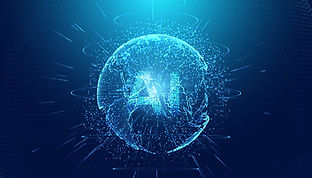.jpg)
The experiment will deploy at least an edge node that allows to create a Digital Twin of the lab and its systems. The main objective is to provide a system with AI/ML models to study approaches to optimize a virtualized intralogistics or production process both from the operational and energy efficiency point of view. The Didactic Factory aims to be a safe environment where novel algorithms and approaches can be tested with the available process data in order to research improvements that could be further optimized later in a real environment.